Natural Language Processing in Planning Documents
Problem, research strategy, and findings: Reading plans is essential for planners for revising current practice and learning new knowledge. However, planners may struggle to find time to read and study lengthy planning documents, especially when there are increasingly more of them, such as emerging climate change plans and urban resilience plans. Recently, natural language processing (NLP) has shown promise in processing big textual data. We ask whether planners can use NLP techniques to more efficiently extract useful and reliable information from planning documents.
By analyzing 78 resilience plans from the 100 Resilient Cities Network, we find that results generated from topic modeling, which is an NLP technique, coincide to a large extent (80%) with those from the conventional content analysis approach. Topic modeling is generally effective and efficient in extracting the main information of plans, while the content analysis approach can find more in-depth details but at the expense of considerable time and effort.
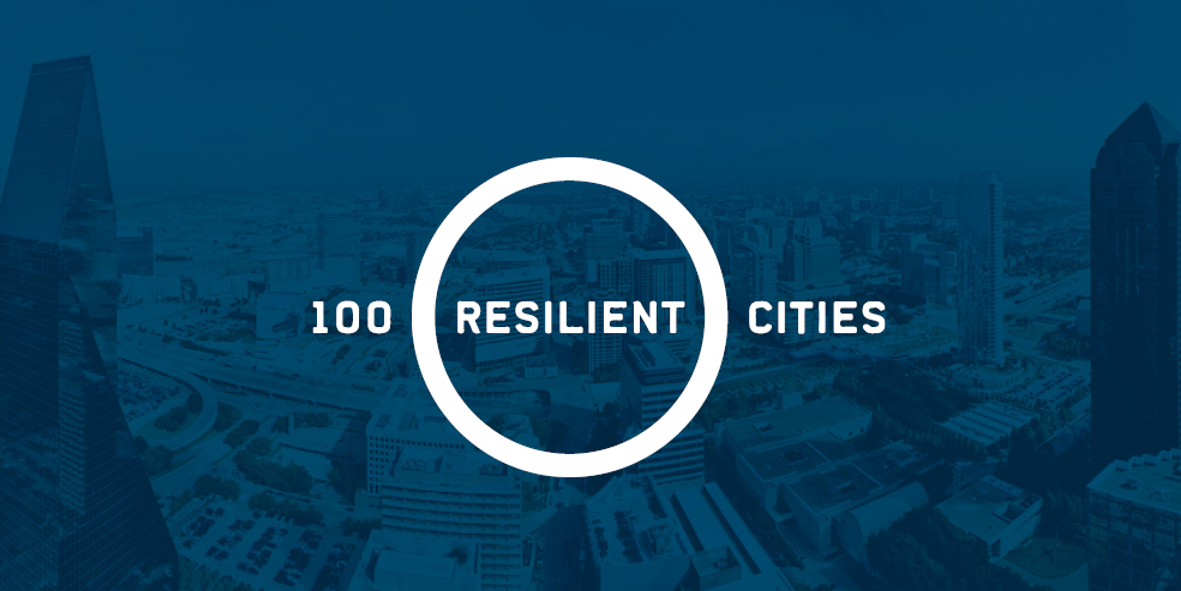
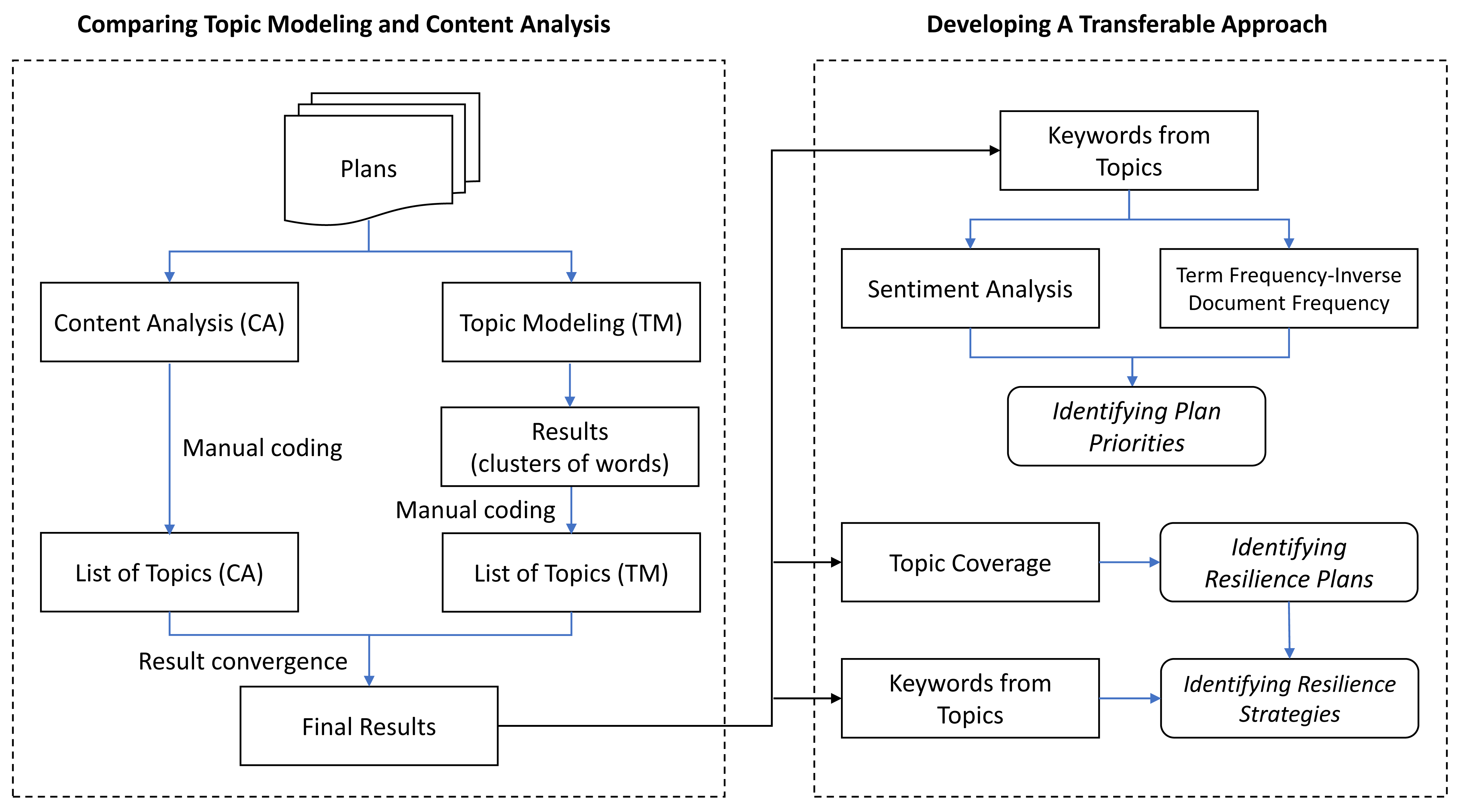