Social media and disaster commmunication
A disaster-resilient city should address social inequity in all its forms. Complete, accurate, and up-to-the-minute situational awareness (SA) can help disaster relief organizations stabilize the dangers and prevent further losses in poor and ethnic minority neighborhoods. However, the lapse of months or years of survey data could lead to biases since retrospective studies are affected by the emotional status at the time of the survey. To address this, we aim to examine the effects of neighborhood equity on SA in a hurricane event using geotagged Twitter data. We adopted the sentiment analysis, convolutional neural network (CNN) model and latent Dirichlet allocation (LDA) model to reflect SA in Hurricane Florence.
The multinomial logit regression model reveals that the tweet originated from a poor neighborhood has a higher probability of being more negative, compared to being neutral. Also, we surprisingly found that the sentiment of a tweet in a black neighborhood could be less likely to be negative. Another novel finding is that black neighborhoods could discuss the hurricane with a positive attitude while poor neighborhoods are more concerned about the work during the hurricane. This study shows that, by incorporating with aggregated sociodemographic data, geotagged Twitter data can also be used to understand disaster SA from the perspective of social equity.
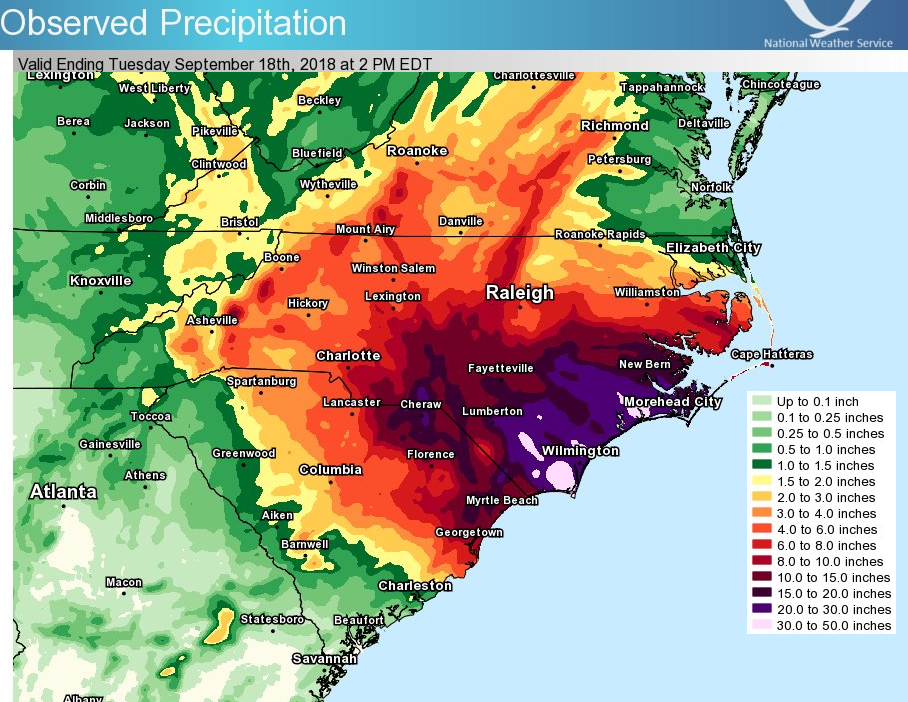
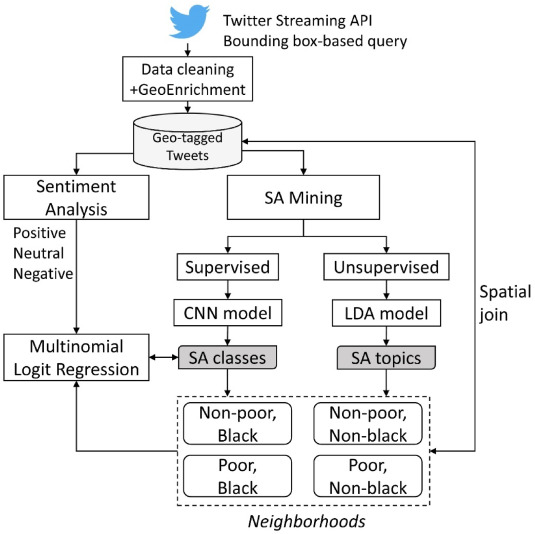
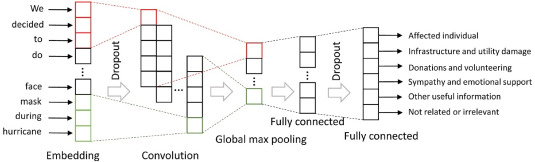
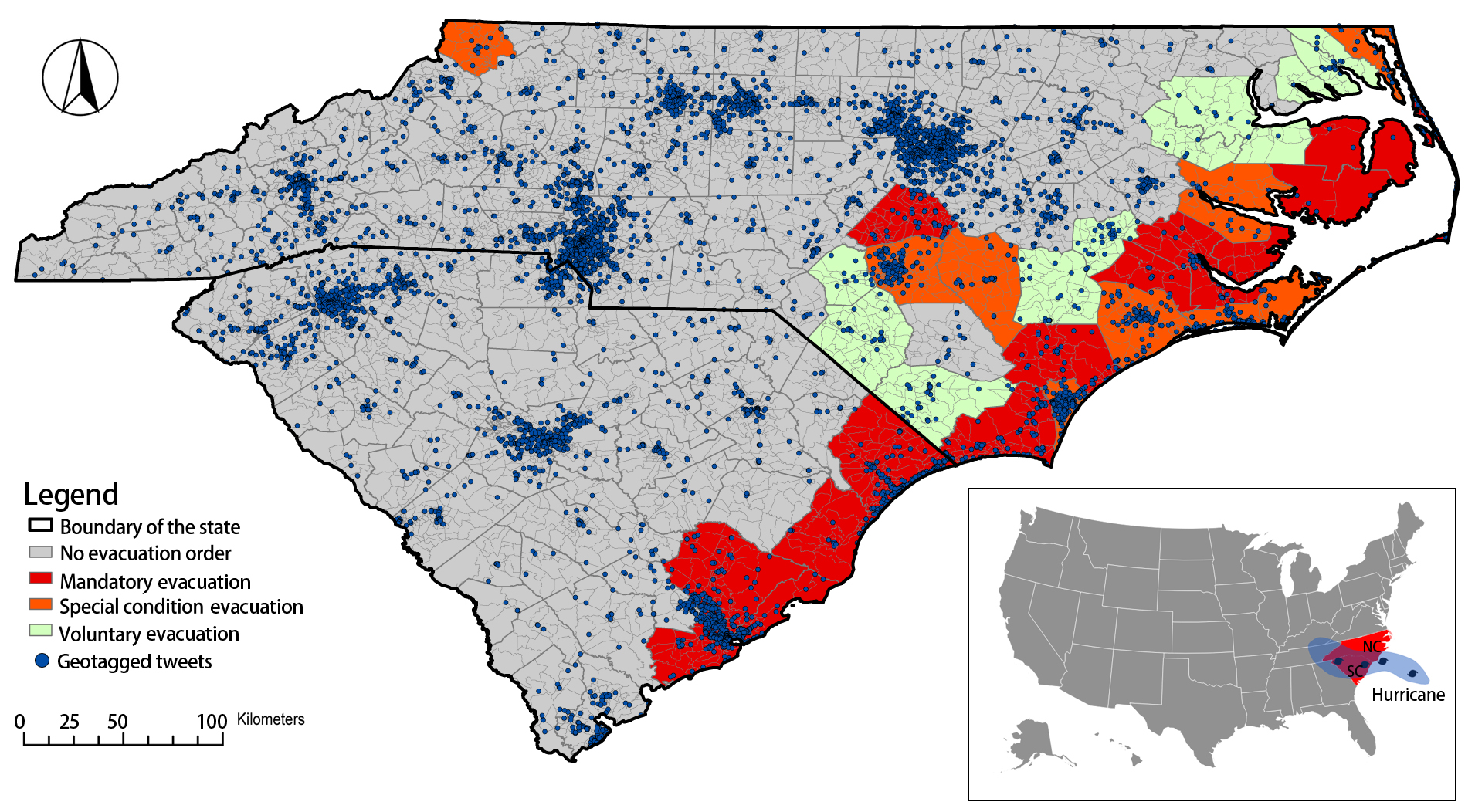