Post-disaster damage assessment
Assessing damage on the ground is a challenging task for humanitarian organizations and disaster managers due to the limited availability of data and methods for processing. As the most commonly adopted data source, remote sensing imagery can only reflect the damage situation on top of a building and fails to present the damage level from the perspective of the human eye.
Recently, an increasing number of Google Street View (GSV) images provide the chance to understand the human's perception of damage on the ground. However, to automatically and quantitatively apply GSV images in damage assessment, two research questions need to be answered: (1) Can deep learning be successfully applied to automate the process of evaluating postdisaster damage using GSV images? (2) Does damage assessment using GSV images provide a different insight, compared with existing approaches, such as remote sensing imagery?
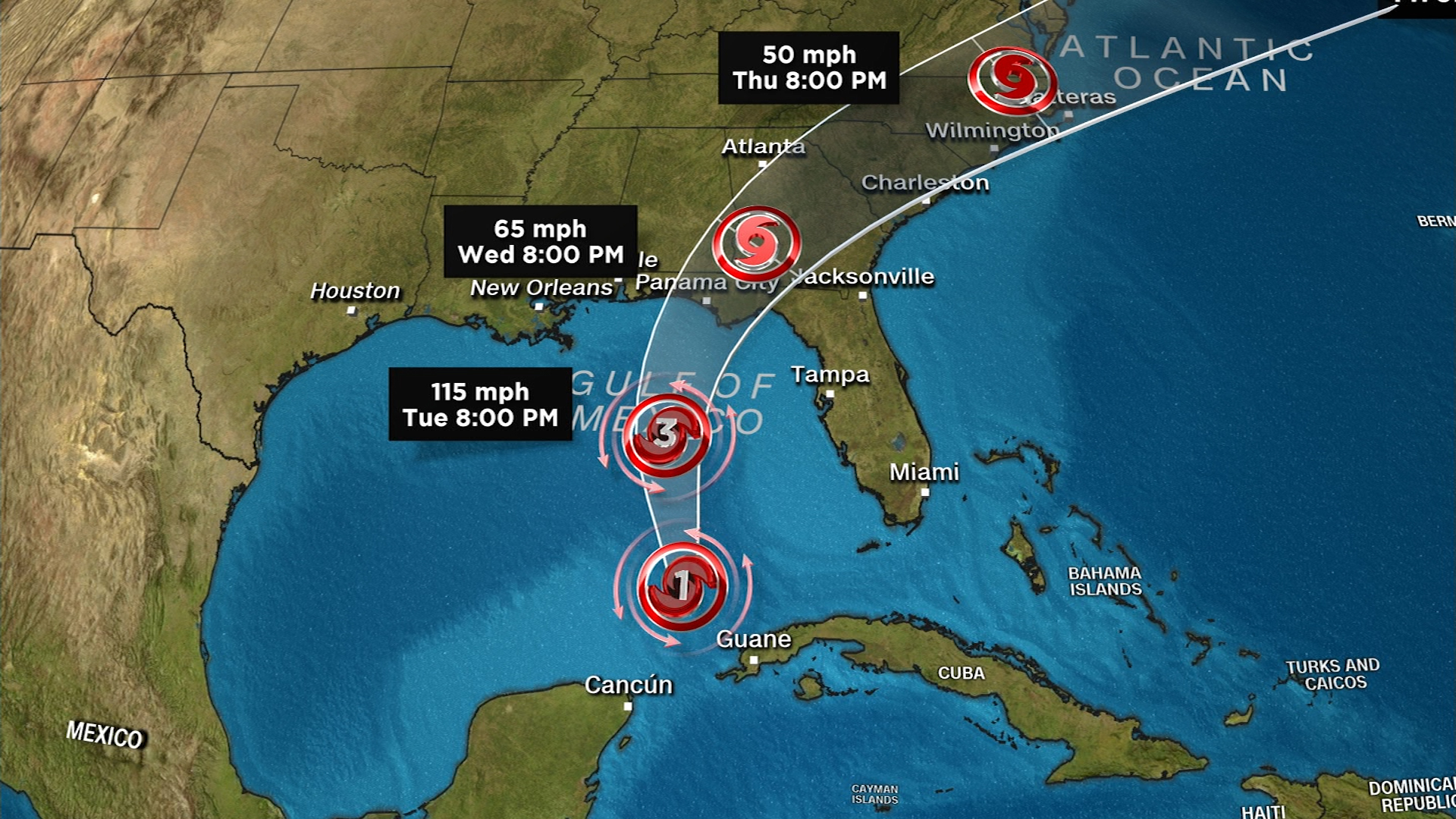
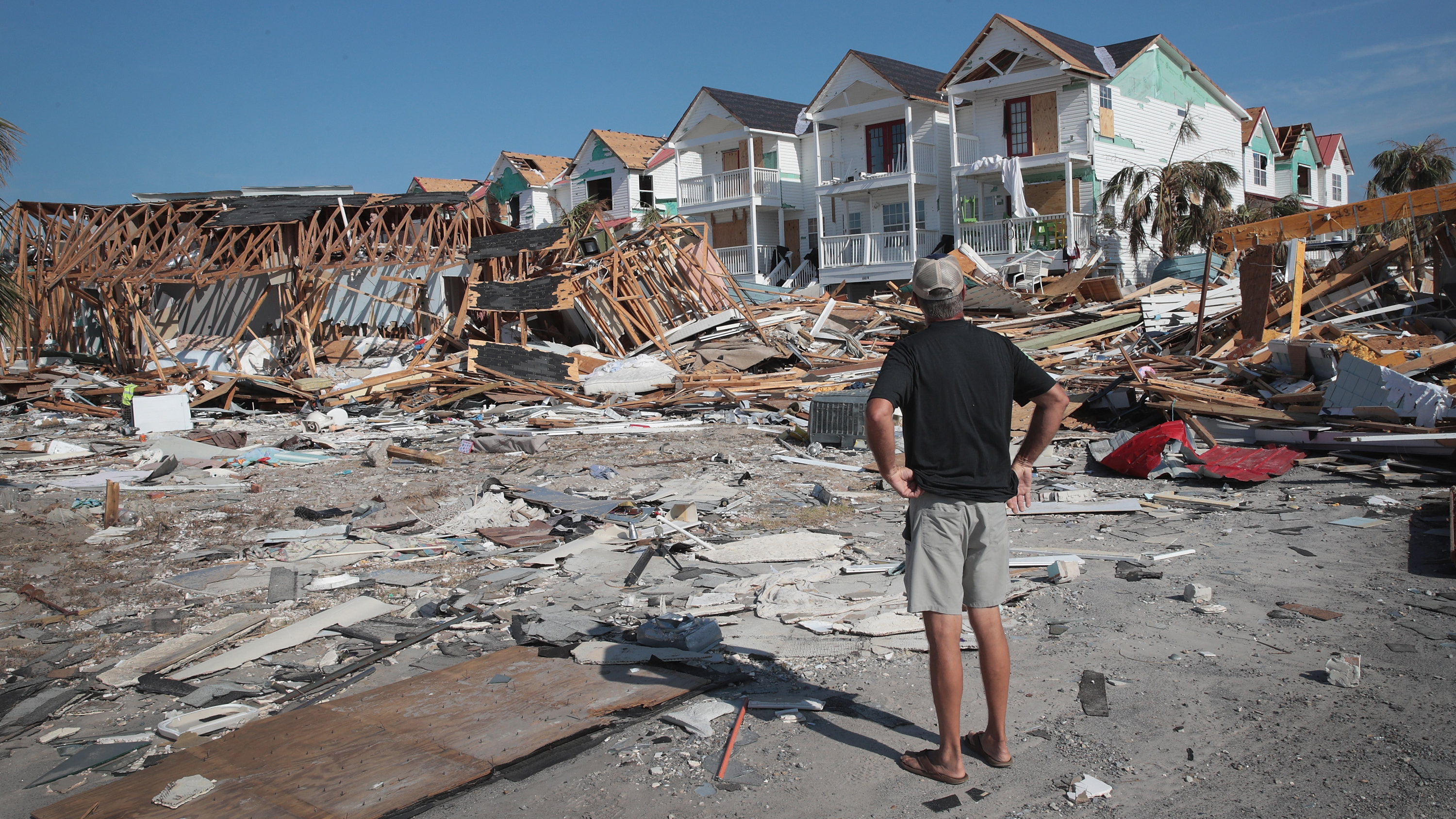
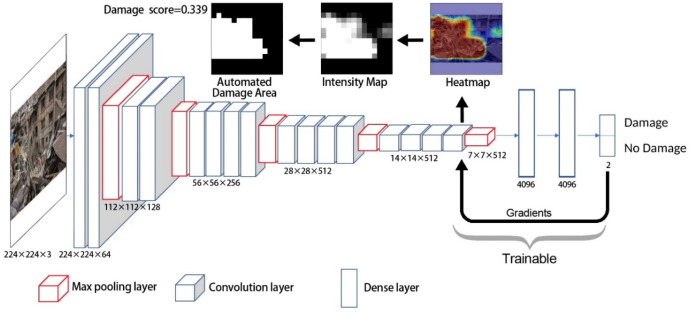
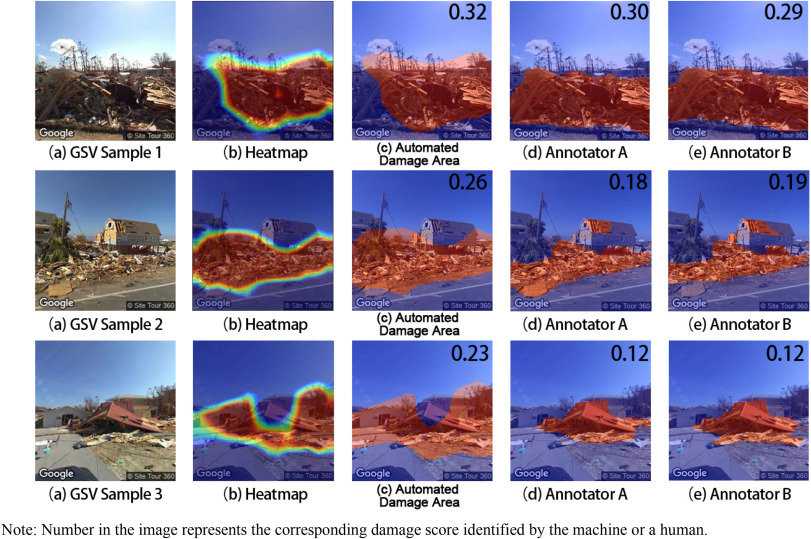